AI systems coming up with biased results - due to the datasets they are trained on
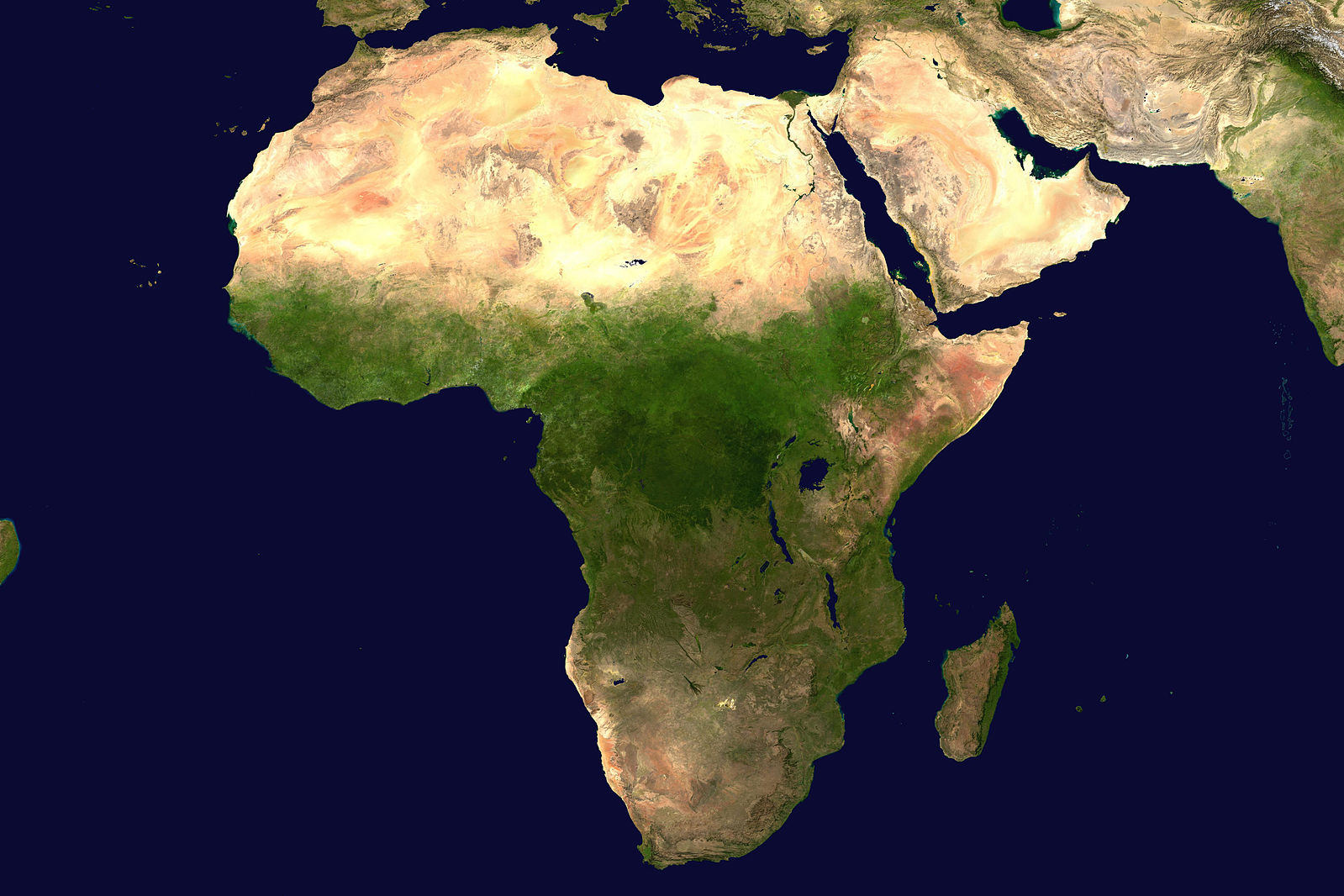
AI systems are producing biased results because they are not being trained on diverse datasets, with little or no representation of African foods and culture, according to a new study.
The study, ‘Addressing Artificial Intelligence Bias through Inclusivity: A Case Study with Nigerian Food Images', will be presented at the first ever Minoritised Life Scientists Future Forum this spring.
Tito Osadebey, from Keele University in the UK, reported back on his findings after creating a Dataset of Nigerian food imagery and attempting to train several deep learning models on the dataset.
“AI systems often show bias against non-Western content due to limited training data. This leads to real-world problems - from facial recognition systems failing to properly identify people with darker skin tones to AI systems being unable to recognise African foods and cultural elements,” he said.
“Our goal is to contribute to tackling bias by primarily creating a dataset of Nigerian food images which can improve AI fairness.”
His interest was sparked while he was working on some personal projects in early 2024, and came across a classification project for foods such as pizza, steak and sushi.
“My curiosity led me to do something similar for Nigerian food but then while searching the internet, I could not find a dataset of Nigerian food. Hence, we identified a significant gap in AI model training - specifically, the lack of diverse, non-Western datasets - which contributes to bias in AI systems,” he says.
“Our study addresses this by creating a Nigerian food image dataset and training deep learning models on it, highlighting the importance of inclusivity in AI development.”
The researchers collected and curated a dataset of 1,546 images representing 10 popular Nigerian dishes. The aim was to collect over 5,000 images but the researchers found that most images were repeated and had to remove them so that the model could generalize well.
“We then trained deep learning models (ResNet-50 and EfficientNet-B0) on this dataset to classify these foods. Our experiments showed that ResNet-50, when trained with a batch size of 16, achieved the highest accuracy (85%) but still struggled with underrepresented food classes, reinforcing the idea that AI performance is strongly tied to the diversity of its training data,” Mr. Osadebey said.
One surprising find was that even with the best-performing model, accuracy varied across food types, with some dishes like banga soup having lower recognition rates. This highlighted a key insight: even within a single cultural dataset, imbalances in representation can still lead to bias, emphasising the need for even more diverse and comprehensive data collection.
“Our findings confirm that AI bias is not just about flawed algorithms—it starts with the data. If AI systems continue to be trained on datasets that overlook certain cultures, they will keep producing biased results,” Mr. Osadebey said.
“This matters because AI is increasingly used in critical areas like healthcare, recruitment, and law enforcement. Ensuring that AI models are trained on diverse datasets is essential for creating fair and accurate technology that serves everyone equally.
“Future research should focus on expanding the dataset to include more African foods and other cultural elements, such as traditional clothing and language processing. Additionally, developing global AI benchmarking standards that require diverse training datasets would be a step toward reducing bias in AI systems.”
The study was led by Tito Osadebey, with contributions from Samuel Oyefusi and Micah Udeogu, who assisted with data collection, model training and evaluation, and documentation.
The Minoritised Life Sciences Future Forum conference (MLSFF) takes place from 31st March to 2nd April 2025 at the ICC Birmingham.
The final date to submit abstracts is 3 March 2025. To apply for a registration fee waiver and/or travel bursary you must apply for these before 28 February 2025. More details can be found here.
To interview Mr Osadebey, please contact [email protected].